LLM Tuning
LLMs Fine-Tuned for Your Business—Not Benchmarks
We specialize in fine-tuning large language models (LLMs) to power mission-critical applications in talent acquisition and cybersecurity. Unlike generic AI models, our custom-tuned systems understand industry-specific terminology, comply with regulatory requirements, and align with unique processes. We employ parameter-efficient fine-tuning (PEFT) techniques like LoRA and QLoRA to adapt models without excessive computational costs. This allows us to create specialized versions of open-source models or enhance proprietary systems while maintaining data privacy.
Our Solution
We develop specialized language models through precision fine-tuning, transforming general-purpose AI into strategic solutions for hiring, security, and industry-specific applications. Our approach goes beyond basic model customization to create systems with decision-making frameworks.
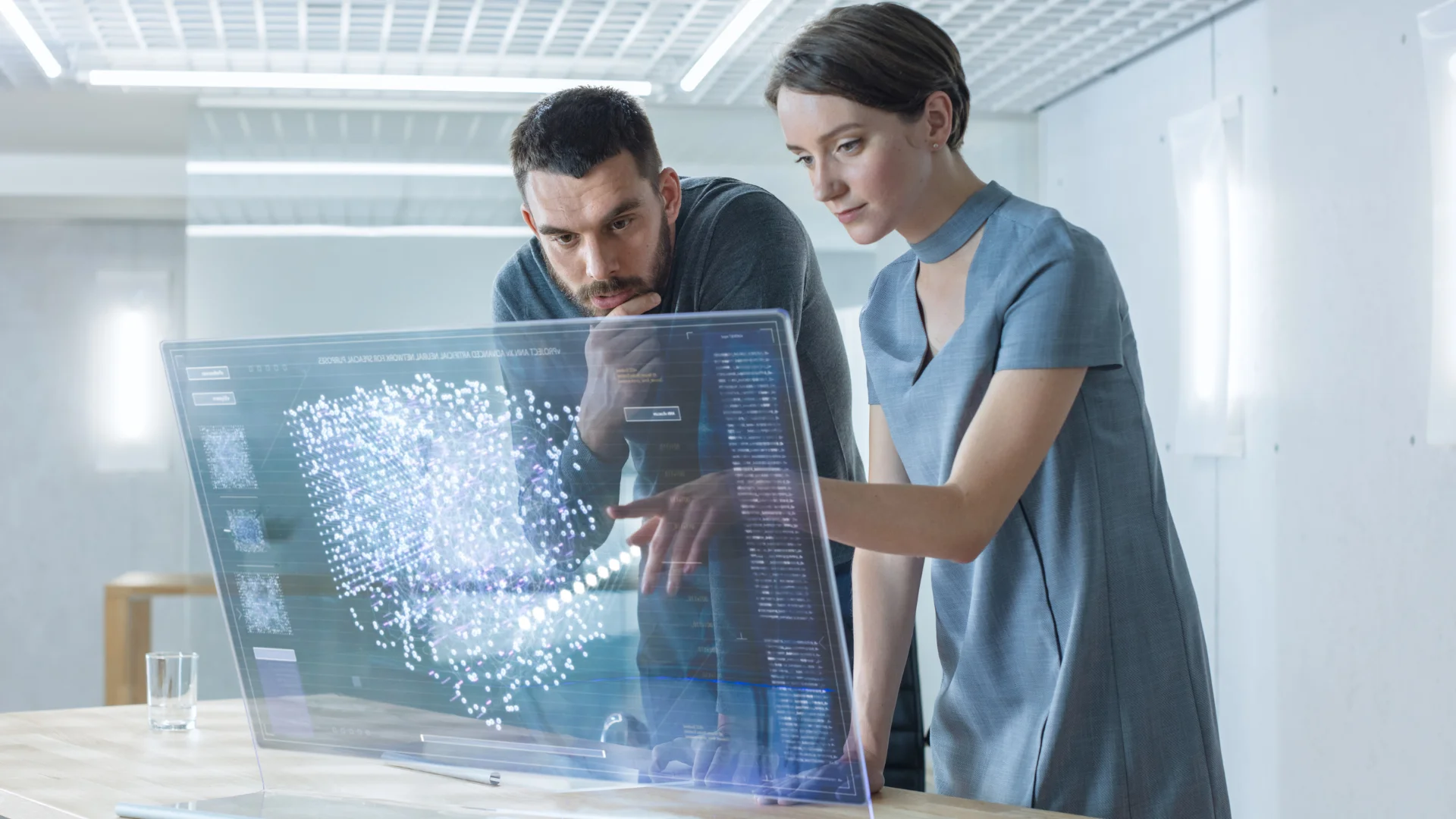
Custom AI Models
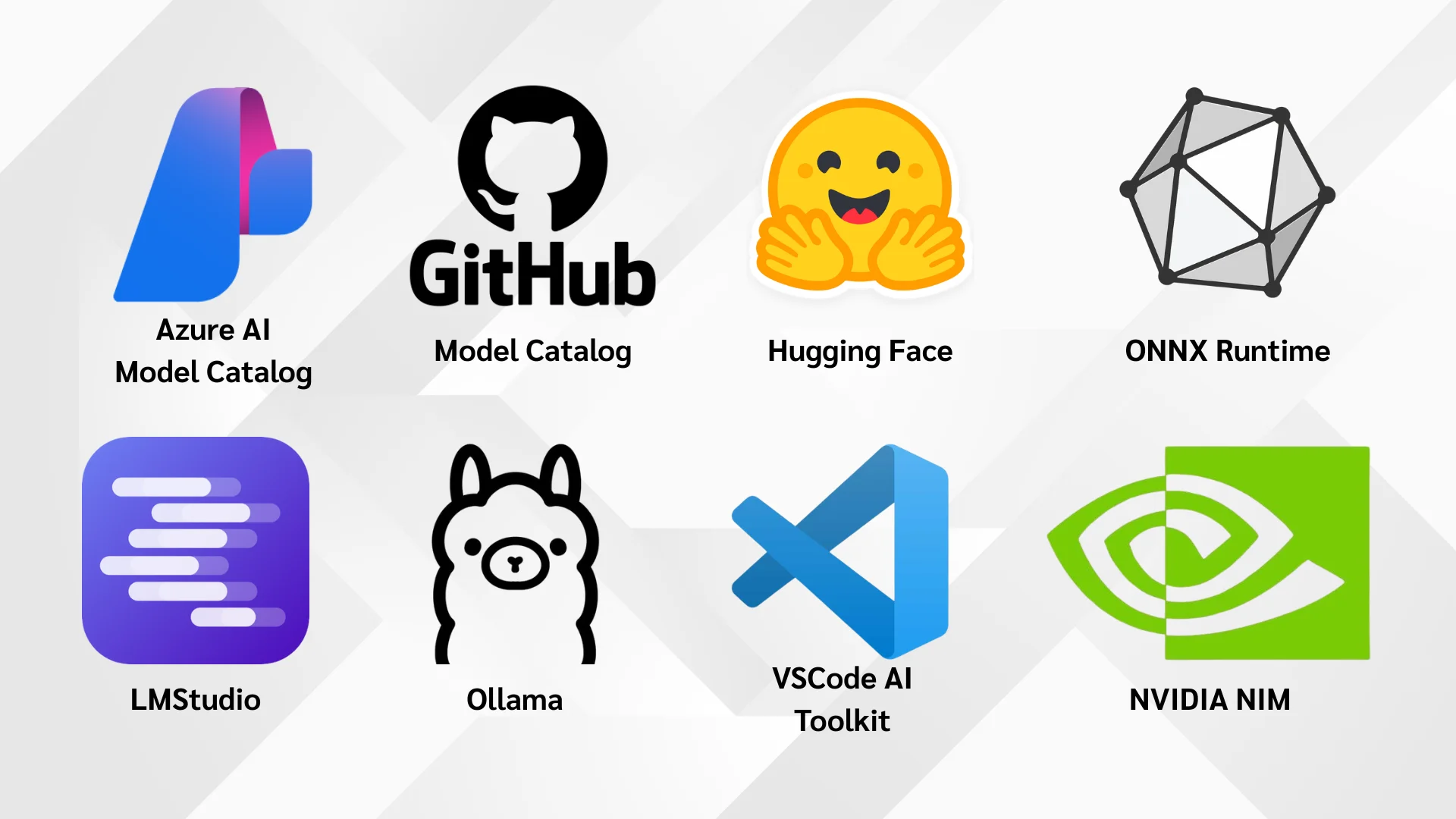
Our recruitment-tuned models analyze candidate materials with human-level comprehension while avoiding the pitfalls of generic AI. We train systems to extract nuanced qualifications from resumes. The tuning process incorporates specific evaluation to ensure alignment with hiring goals. We implement multiple safeguards to maintain EEOC and GDPR compliance, including bias testing protocols and explanation features for all AI-generated assessments.
How It Works
Our tuning process begins with a collaborative workshop to map requirements for model capabilities. Data scientists then curate and annotate training sets that reflect real-world usage scenarios by applying techniques like parameter fine-tuning to maximize impact while minimizing computational costs. For most implementations, we recommend LoRA (Low-Rank Adaptation) or QLoRA (Quantized LoRA) approaches by adjusting model parameters.
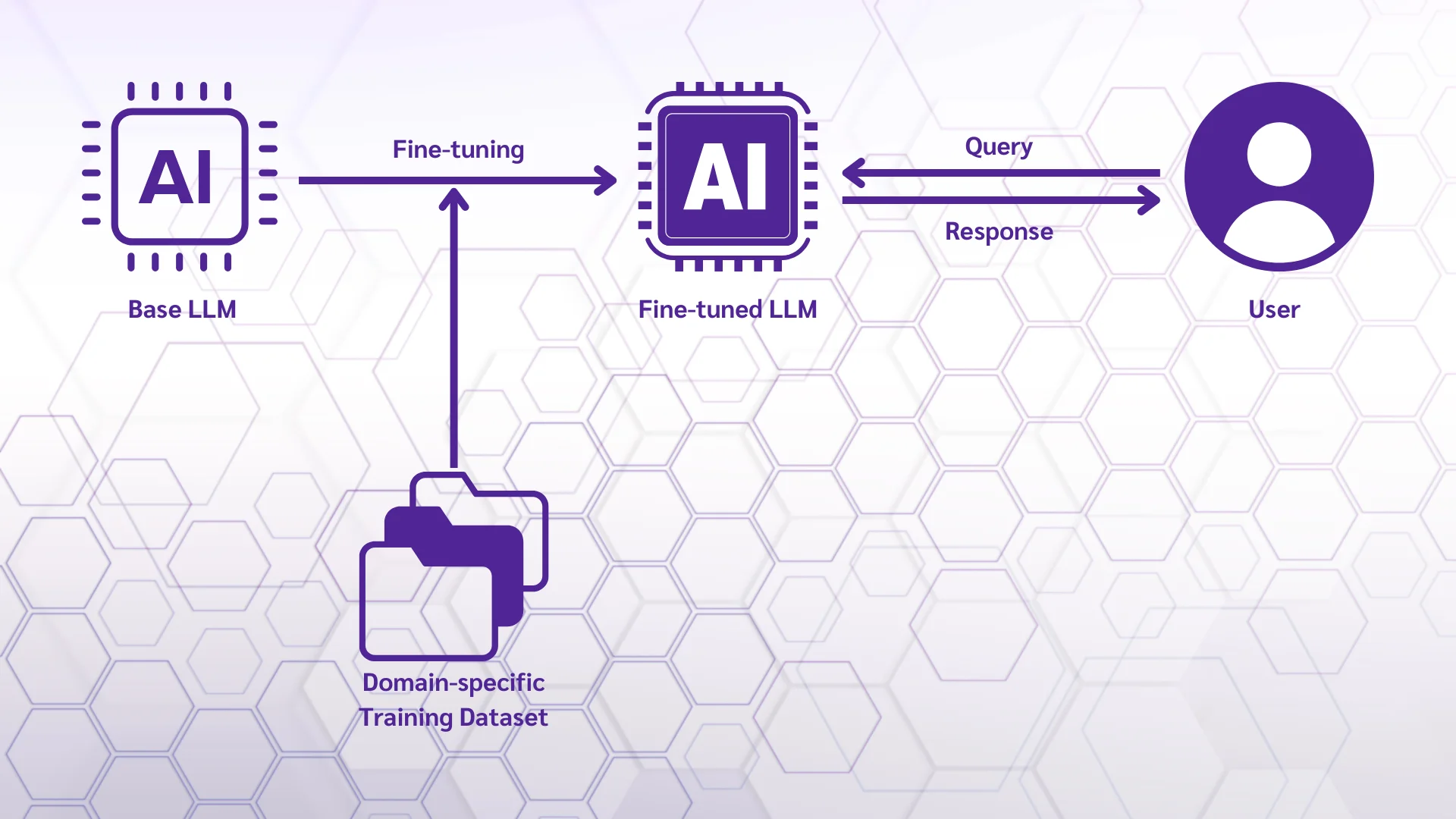
AI Implementation
We guide clients through a structured deployment process that emphasizes responsible AI use. The typical engagement includes capability assessment, pilot testing with real users, and the establishment of monitoring protocols to detect model drift. All tuned models ship with documentation covering their training data sources, known limitations, and recommended guardrails. For high-stakes applications, we build in approval workflows that require human validation before acting on critical model outputs.